Share this
AI vs. ML: What's the Difference?
by Kory Underdown on Apr 27, 2023 1:00:00 PM
Since an MIT researcher first coined the term in the 1950s, artificial intelligence has exploded in popularity. Today, AI powers everything from coffee machines and mattresses to surgical robots and driverless trucks. Its many applications prove that technology can mimic—and enhance—the human experience.
You’ll often hear the terms artificial intelligence and machine learning used interchangeably, but AI and ML, while closely interrelated, are not the same concept. AI is a broad label that defines a host of technological capabilities and systems. ML, on the other hand, is a subset of AI with a much more narrow scope.
WHAT IS ARTIFICIAL INTELLIGENCE?
The field of AI encompasses technology that can perform tasks that have traditionally required human intelligence. If a machine can reason, problem-solve, make decisions, and learn new things, it fits into this category. The scope is essentially limitless.
Today, AI is used in a wide variety of applications. Manufacturers use AI to program and control robots in order to automate physical processes. Companies are using AI to scan text and images to pull out relevant information for study or analysis. If you have a smartphone that recognizes your face—that’s a form of AI.
WHAT IS MACHINE LEARNING?
Whereas AI is a broad concept, ML is a specific application of that concept. Machine learning is a type of AI that makes it possible for computers to learn from experience as opposed to direct human programming. Importantly, ML capabilities are limited to performing tasks that the system has specifically been trained to do, and ML’s scope is therefore much more focused.
ML lets you glean new information from existing data, and it’s primarily used to uncover complex patterns, predict outcomes, and detect anomalies. Google’s search tool uses ML algorithms to find relevant content for users by studying their search behaviors. LinkedIn leverages machine learning to provide recommendations and supercharge its talent search model. Social media platforms turn to ML to suggest potential connections, banks use the technology to detect fraud, e-commerce sites improve their recommendations, and cybersecurity providers enhance spam and malware filtering using ML.
THE DIFFERENCE BETWEEN AI AND ML
At its most basic, ML gives machines knowledge, and AI gives machines the ability to apply that knowledge to solve complex problems. ML can help grow the knowledge base of AI without the need for human inputs or teachings.
Again, the difference here is primarily one of scope: AI is an umbrella term that includes ML, but is often used to describe applications that use machines to do the work of humans. By contrast, ML focuses on teaching computers and machines new information without human help.
POTENTIAL DOWNSIDES OF AI AND ML
While companies across industries are investing more and more into AI and ML to help their businesses, these technologies have downsides that are important to consider.
The first downside will be familiar to anyone operating in a bleeding edge space in the tech world: AI and ML can be unpredictable. These are new technologies being used in new applications, and their ability to disrupt older ways of doing things can be just as much of a problem for businesses as it can be a solution.
The novelty of AI and ML also means that there are—at present—relatively few people that understand these systems forwards and backwards. This can make it difficult for companies looking to take advantage of AI and ML to reliably control them.
As AI applications streamline processes, they also run the risk of putting people out of work. These applications can also make workers excessively reliant on technology, leading to skill atrophy and a lesser ability to problem solve when issues arise.
Finally, without careful implementation, AI applications can create data privacy problems for businesses and individuals. AI solutions typically require organizations to input massive amounts of personal data—the more data, the better the solution. As a result, organizations and individuals may have to give up a right to privacy in order for AI to work effectively.
AI applications that are hosted on public networks can also expose sensitive data to outsiders and malicious actors. Networked AI applications that rely on private data (including a company’s proprietary information) can expose organizations to new risks of data breaches.
The advantage of ML
In many cases, ML can be a better option than AI because it lacks many of the downsides we just explored. Because ML is more tightly focused on improving the knowledge base and efficiency of computers, it doesn’t necessarily produce the same data privacy risks as AI.
And because the scope of ML is more narrow than that of AI, there’s less room for unpredictable or negative outcomes to occur. Businesses looking to mitigate their exposure to risk should be more comfortable with ML technologies rather than the broader umbrella of AI applications.
SEEKING A BALANCE IN BUSINESS
AI and ML are natural partners. While researchers are finding new ways to use AI to work smarter, ML is making computers and AI systems themselves smarter.
Unfortunately, AI in its current state comes with a number of significant downsides which may make it too risky or unreliable a technology for many businesses to use. On the other hand, ML can be employed with greater control and focus, reducing risk while maximizing the benefits of using machines to increase productivity.
DNSFilter uses ML with our Webshrinker product, which allows us to categorize websites a full seven days sooner than more traditional threat feeds. DNSFilter’s Machine Learning is the Goldilocks solution for this digital moment: Fast without being unpredictable (like AI can be). Instead, ML is just right. Want to see for yourself? Try DNSFilter free for 14 days.
Share this
Categories
- Featured (267)
- Protective DNS (23)
- IT (15)
- IndyCar (9)
- Content Filtering (8)
- AI (7)
- Cybersecurity Brief (7)
- IT Challenges (7)
- Public Wi-Fi (7)
- Deep Dive (6)
- Malware (4)
- Roaming Client (4)
- Team (4)
- Compare (3)
- MSP (3)
- Machine Learning (3)
- Phishing (3)
- Ransomware (3)
- Staying Ahead of Cyber Threats (3)
- Tech (3)
- Anycast (2)
- Events (2)
- Tech Stack (2)
- Secure Web Gateway (1)
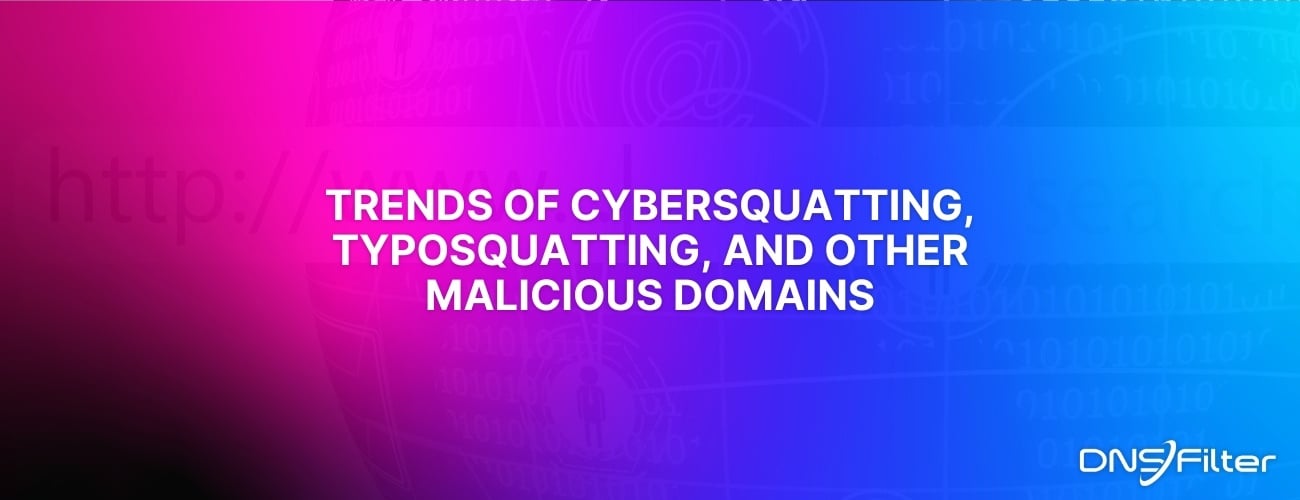
The Growing Threat of Malicious Domains in Cybersecurity
As cybercriminals continue to evolve their tactics, domain-based attacks like cybersquatting, typosquatting, and other malicious domains have become a significant threat to businesses and individuals alike. These attacks are designed to exploit trust, impersonate brands, and mislead users into handing over sensitive information—often resulting in financial losses, data breaches, and reputat...
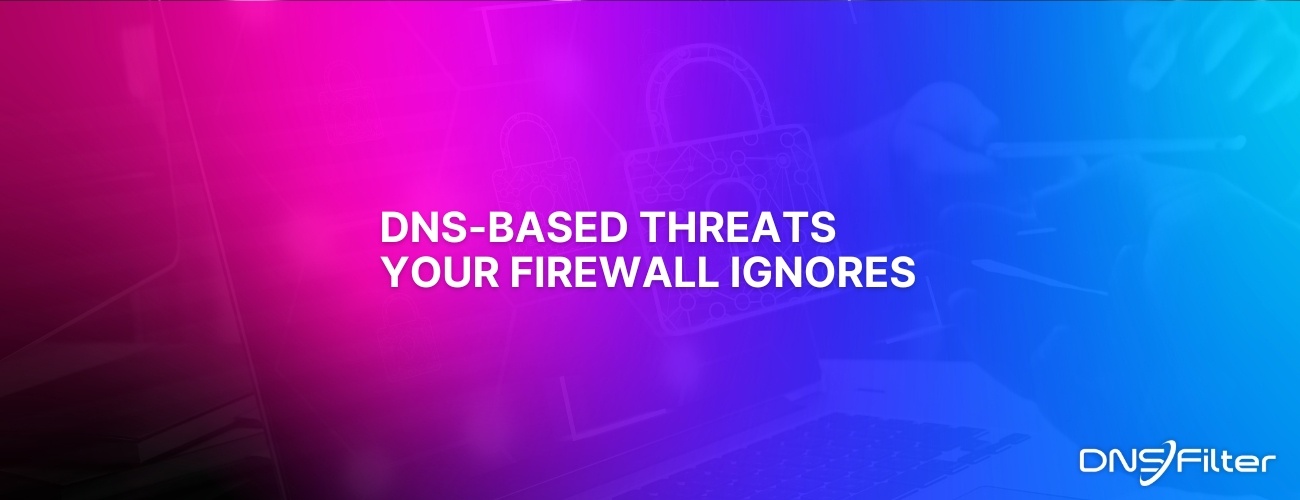
Your firewall is working hard… but not smart. And cybercriminals love that.
Like a bouncer at the club with a clipboard—great at stopping the obvious troublemakers that aren’t on the list, but completely oblivious to unknown threats. They excel at blocking unauthorized access through known ports and protocols, but they often overlook a critical vulnerability: DNS traffic and what’s on the other side of a link. This oversight allows cybercrimina...
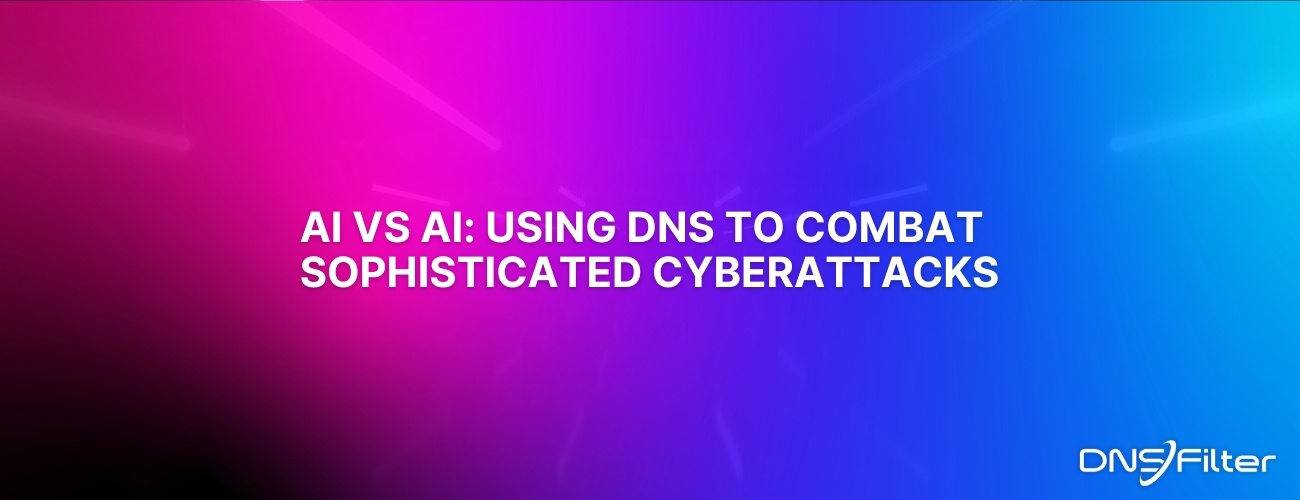
Introduction: The AI Cybersecurity Arms Race
Artificial intelligence (AI) has transformed the cybersecurity landscape—both for defenders and attackers. While AI-powered cybersecurity solutions offer advanced threat detection, AI-driven cybercrime is evolving at an alarming rate, automating attacks that are more sophisticated, evasive, and dangerous.